Multi-Level Indexing
In this lesson, we are going to explore techniques to permit advanced data exploration and analysis using Python. We will focus on the Pandas library, focusing on real-life case scenarios to help you to better understand how data can be processed using Pandas.
In particular, we will explore the concept of tidy datasets, the concept of multi-index, and its impact on real datasets, and the concept of concatenating and merging different Pandas objects, with a focus on DataFrames. We’ll look at how to transform a DataFrame, and how to plot results with Pandas.
If you have any feedback relating to this lesson, feel free to reach out to us at support@cloudacademy.com.
Learning Objectives
- Learn what a tidy dataset is and how to tidy data
- Merge and concatenate tidy data
- Learn about multi-level indexing and how to use it
- Transform datasets using advanced data reshape operations with Pandas
- Plot results with Pandas
Intended Audience
This lesson is intended for data scientists, data engineers, or anyone looking to perform data exploration and analysis with Pandas.
Prerequisites
To get the most out of this lesson, you should have some knowledge of Python and the Pandas library. If you want to brush up on Pandas, we recommend taking our Working with Pandas lesson.
Resources
The GitHub repo for this lesson can be found here.
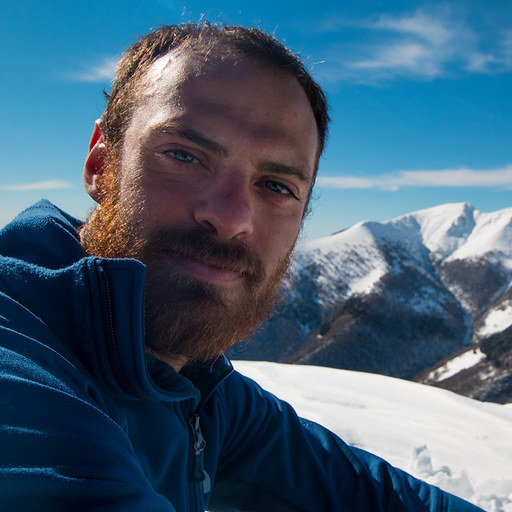
Andrea is a Data Scientist at Cloud Academy. He is passionate about statistical modeling and machine learning algorithms, especially for solving business tasks.
He holds a PhD in Statistics, and he has published in several peer-reviewed academic journals. He is also the author of the book Applied Machine Learning with Python.