Distributed Machine Learning
This training lesson begins with an introduction to the concepts of Distributed Machine Learning. We'll discuss the reasons as to why and when you should consider training your machine learning model within a distributed environment.
Apache Spark
We’ll introduce you to Apache Spark and how it can be used to perform machine learning both at scale and speed. Apache Spark is an open-source cluster-computing framework.
Amazon Elastic Map Reduce
We’ll introduce you to Amazon’s Elastic MapReduce service, or EMR for short. EMR provides a managed Hadoop framework that makes it easy, fast, and cost-effective to process vast amounts of data. EMR can be easily configured to host Apache Spark.
Spark MLlib
We’ll introduce you to MLlib which is Spark’s machine learning module. We’ll discuss how MLlib can be used to perform various machine learning tasks. For this lesson, we'll focus our attention on decision trees as a machine learning method which the MLlib module supports. A decision tree is a type of supervised machine learning algorithm used often for classification problems.
AWS Glue
We’ll introduce you to AWS Glue. AWS Glue is a fully managed extract, transform, and load service, ETL for short. We’ll show you how AWS Glue can be used to prepare our datasets before they are used to train our machine learning models.
Demonstration
Finally, we’ll show you how to use each of the aforementioned services together to launch an EMR cluster configured and pre-installed with Apache Spark for the purpose of training a machine learning model using a decision tree. This demonstration will provide an end-to-end solution that provides machine learning predictive capabilities.
Intended Audience
The intended audience for this lesson includes:
- Data scientists and/or data analysts
- Anyone interested in learning and performing distributed machine learning, or machine learning at scale
- Anyone with an interest in Apache Spark and/or Amazon Elastic MapReduce
Learning Objectives
By completing this lesson, you will:
- Understand what Distributed machine learning is and what it offers
- Understand the benefits of Apache Spark and Elastic MapReduce
- Understand Spark MLlib as machine learning framework
- Create your own distributed machine learning environment consisting of Apache Spark, MLlib, and Elastic MapReduce.
- Understand how to use AWS Glue to perform ETL on your datasets in preparation for training a your machine learning model
- Know how to operate and execute a Zeppelin notebook, resulting in job submission to your Spark cluster
- Understand what a machine learning Decision Tree is and how to code one using MLlib
Prerequisites
The following prerequisites will be both useful and helpful for this lesson:
- A background in statistics or probability
- Basic understanding of data analytics
- General development and coding experience
- AWS VPC networking and IAM security experience (for the demonstrations)
Lesson Agenda
The agenda for the remainder of this lesson is as follows:
- We’ll discuss what Distributed Machine Learning is and when and why you might consider using it
- We’ll review the Apache Spark application, and its MLlib machine learning module
- We’ll review the Elastic MapReduce service
- We’ll provide an understanding what a Decision Tree is - and what types of analytical problems it is suited towards
- We’ll review the basics of using Apache Zeppelin notebooks - which can be used for interactive machine learning sessions
- We’ll review AWS Glue. We’ll show you how you can use AWS Glue to perform ETL to prepare our datasets for ingestion into a machine learning pipeline.
- Finally - We’ll present a demonstration of a fully functional distributed machine learning environment implemented using Spark running on top of an EMR cluster
Feedback
If you have thoughts or suggestions for this lesson, please contact Cloud Academy at support@cloudacademy.com.
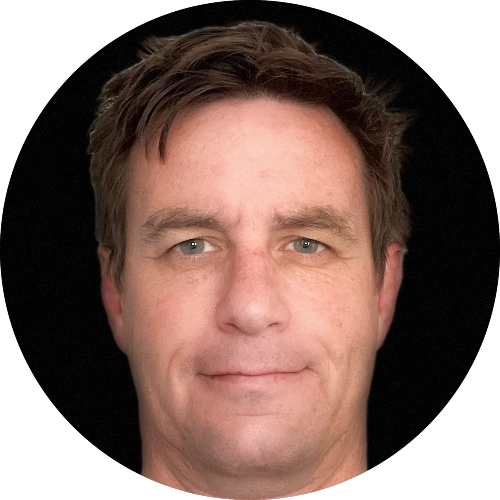
Jeremy is a Content Lead Architect and DevOps SME here at Cloud Academy where he specializes in developing DevOps technical training documentation.
He has a strong background in software engineering, and has been coding with various languages, frameworks, and systems for the past 25+ years. In recent times, Jeremy has been focused on DevOps, Cloud (AWS, Azure, GCP), Security, Kubernetes, and Machine Learning.
Jeremy holds professional certifications for AWS, Azure, GCP, Terraform, Kubernetes (CKA, CKAD, CKS).