Training the Model
This lesson takes an introductory look at using the SageMaker platform, specifically within the context of preparing data, building and deploying machine learning models.
During this lesson, you'll gain a practical understanding of the steps required to build and deploy these models along with learning how SageMaker can simplify this process by handling a lot of the heavy lifting both on the model management side, data manipulation side, and other general quality of life tools.
If you have any feedback relating to this lesson, feel free to contact us at support@cloudacademy.com.
Learning Objectives
- Obtain a foundational understanding of SageMaker
- Prepare data for use in a machine learning project
- Build, train, and deploy a machine learning model using SageMaker
Intended Audience
This lesson is ideal for data scientists, data engineers, or anyone who wants to get started with Amazon SageMaker.
Prerequisites
To get the most out of this lesson, you should have some experience with data engineering and machine learning concepts, as well as familiarity with the AWS platform.
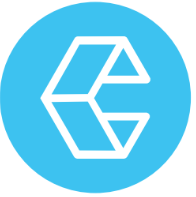
Calculated Systems was founded by experts in Hadoop, Google Cloud and AWS. Calculated Systems enables code-free capture, mapping and transformation of data in the cloud based on Apache NiFi, an open source project originally developed within the NSA. Calculated Systems accelerates time to market for new innovations while maintaining data integrity. With cloud automation tools, deep industry expertise, and experience productionalizing workloads development cycles are cut down to a fraction of their normal time. The ability to quickly develop large scale data ingestion and processing decreases the risk companies face in long development cycles. Calculated Systems is one of the industry leaders in Big Data transformation and education of these complex technologies.