Bayesian probability
Beginner
11m 15s
784
3.5/5
This lesson explores the topic of probability and statistics, including various mathematical approaches and some different interpretations of probability. The lesson starts off with an introduction to probability, before moving on to cover the topics of Bayesian probability, Frequentist probability, statistics, probability distribution and normal distribution.
About the Author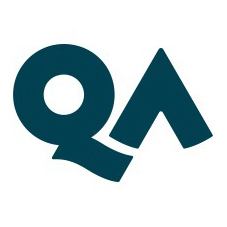
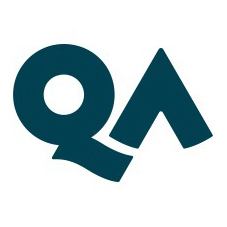
QA, opens in a new tabTraining Provider
Students51,132
Labs185
Courses2,497
Learning paths47
A world-leading tech and digital skills organization, we help many of the world’s leading companies to build their tech and digital capabilities via our range of world-class training courses, reskilling bootcamps, work-based learning programs, and apprenticeships. We also create bespoke solutions, blending elements to meet specific client needs.