SageMaker Studio - Getting Started with Data Wrangler
Get started with the latest Amazon SageMaker services — Data Wrangler, Data Pipeline and Feature Store services — released at re:Invent Dec 2020. We also learn about the SageMaker Ground Truth and how that can help us sort and label data.
Get a head start in machine learning by learning how these services can reduce the effort and time required for you to load and prepare data sets for analysis and modeling. Data scientists will often spend 70% or more of their time cleaning, preparing, and wrangling their data into a state where it’s suitable to train machine learning algorithms against the data. It’s a lot of work, and these new SageMaker services provides an easier way.
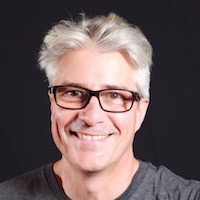
Andrew is fanatical about helping business teams gain the maximum ROI possible from adopting, using, and optimizing Public Cloud Services. Having built 70+ Cloud Academy courses, Andrew has helped over 50,000 students master cloud computing by sharing the skills and experiences he gained during 20+ years leading digital teams in code and consulting. Before joining Cloud Academy, Andrew worked for AWS and for AWS technology partners Ooyala and Adobe.