Overview
Azure Data Lake Storage Gen2 (ADLS) is a cloud-based repository for both structured and unstructured data. For example, you could use it to store everything from documents to images to social media streams.
Data Lake Storage Gen2 is built on top of Blob Storage. This gives you the best of both worlds. Blob Storage provides great features like high availability and lifecycle management at a very low cost. Data Lake Storage provides additional features, including hierarchical storage, fine-grained security, and compatibility with Hadoop.
The most effective way to do big data processing on Azure is to store your data in ADLS and then process it using Spark (which is essentially a faster version of Hadoop) on Azure Databricks.
In this lesson, you will follow hands-on examples to import data into ADLS and then securely access it and analyze it using Azure Databricks. You will also learn how to monitor and optimize your Data Lake Storage.
Learning Objectives
- Get data into Azure Data Lake Storage (ADLS)
- Use six layers of security to protect data in ADLS
- Use Azure Databricks to process data in ADLS
- Monitor and optimize the performance of your data lakes
Intended Audience
- Anyone interested in Azure’s big data analytics services
Prerequisites
- Experience with Azure Databricks
- Microsoft Azure account recommended (sign up for free trial at https://azure.microsoft.com/free if you don’t have an account)
Resources
The GitHub repository for this lesson is at https://github.com/cloudacademy/azure-data-lake-gen2.
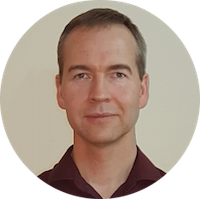
Guy launched his first training website in 1995 and he's been helping people learn IT technologies ever since. He has been a sysadmin, instructor, sales engineer, IT manager, and entrepreneur. In his most recent venture, he founded and led a cloud-based training infrastructure company that provided virtual labs for some of the largest software vendors in the world. Guy’s passion is making complex technology easy to understand. His activities outside of work have included riding an elephant and skydiving (although not at the same time).