Blended learning and learning behavioral patterns were key areas of discussion during this year’s Learning Analytics & Knowledge conference (LAK20). At Cloud Academy, we’re very interested in all things learning and eager to follow the field as it evolves proposing new concepts and technologies.
This year, we had a chance to attend the LAK20 conference, looking for inspiration to advance our technology, as well as proposing our own contribution to the field. LAK targets the intersection of learning and technology, focusing on topics like educational data mining, learning behavior analysis, blending learning techniques, assessment and personalization technologies, and related artificial intelligence techniques, gathering experts from many fields.
Due to the COVID-19 pandemic, this year’s 10th edition LAK20 was held as a fully remote one, deviating from the originally planned Frankfurt location. Here’s my view on just a few of the main topics of the conference.
A successful remote conference
As mentioned, the health emergency forced the conference to move to a fully virtual format. This undeniably had an impact on how participants could experience the event, but the organizers did a spectacular job arranging a fully virtual solution in a ridiculously short time. Kudos!
Talks were spread over two parallel sessions, going from 9 am to late afternoon/evening (Frankfurt time) each of the three conference days. Each talk was allocated a 30-minute slot (the same for short/full papers and practitioner contributions), which in most cases resulted in a 20-25 minute presentation plus a Q&A for the remaining time.
This was my first online conference, and I can say I enjoyed it. The opportunities of an in-person conference are difficult to replicate online, but virtual has its one advantages, particularly in terms of traveling and time saved. I might describe the pace of talks as “intense,” but again I think the organizers found a good solution, accounting for an audience that spanned several time zones at once.
Learning how the learners learn
Many talks at LAK20 focused on the properties of the learning process and how they differ from student to student. This kind of analysis relies on concepts like learning tactics (low-level sequential patterns of learning actions, like taking a quiz or watching a lecture video) and strategies (high-level patterns of learning tactics). Unsupervised AI techniques allow to detect behavioral archetypes from these student activities and can be correlated to their academic results to identify the most effective learning patterns for each student.
Other talks approached learning analysis more interactively, proposing dashboards within several contexts. They can be used by teachers to monitor students’ progress, or the student themselves to provide a sense of control on their own learning. They can allow a teacher to actively explore aspects of her interest, or instead of being alerted when learning anomalies are detected and need correction. Automated dashboards were also proposed, providing an optimal view over the most interesting patterns by using deviation metrics like KL-divergence to drill-down on the most interesting data.
Blended learning
Such interest in monitoring and pattern discovery directly relates to the availability of data around students learning online and, increasingly so, in the classroom. Blended learning was mentioned by many works and appeared as one of the hottest topics at the conference. Once more, this highlights how learning is changing the way it is delivered and blended learning is expected to become the “normal way.” Take a look at this interesting article on blended learning from our friends at QA, Mixing it Up: The Future of Learning is Blended.
Mining language
Something we’re very interested in at Cloud Academy is how we can extract useful information from textual data, such as how to assemble content in meaningful ways. As a matter of fact, Natural Language Processing (NLP) had its share of talks at LAK20.
As an example, consider the activity of students on a Massive Open Online Course (MOOC) forum. By applying text analysis techniques, it is possible to monitor the concepts they discuss online when collaborating to solve an assignment or expressing their feelings and concerns about the course. Methods like text classification can be mixed with psychological and educational notions like teaching, social, and cognitive presence to automatically categorize student interactions and analyze them both. This can be done in the classroom and at the individual level to obtain an overview of the student’s reception of the course, the problems to address, and the improvement opportunities.
Another topic of interest was the extraction of numerical properties from educational content — like the difficulty of exam questions — using machine learning. Several very interesting approaches were proposed aiming at this, including the experiments conducted by the Politecnico di Milano in collaboration with Cloud Academy. In this article, we calibrate new questions using Natural Language Processing (NLP). You can understand the technology behind our questions in the Skill Assessment video below.
Final thoughts
The format of the conference was quite intense but very focused and efficient one. Networking was not very easy, but still, exposure to a wide spectrum of research groups and topics was possible. Perhaps due to the format, I was not able to assess the role and prevalence of industry participants, but I appreciated the presence of practitioner contributions.
The talks highlighted several very relevant trends, both in terms of techniques (behavioral patterns extraction, NLP, dashboards, process mining, etc.) and educational themes (blended learning, collaborative learning, data privacy issues, etc.) — definitely, aspects to keep an eye on.
I’m eager to see what the new LAK edition will look like, presumably in person once again, in sunny California in 2021.
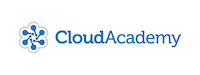
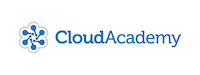