Kinesis Family
Please note: this course has been removed from our Training Library and replaced with the Data Analytics Concepts course.
In this course, we will explore the Analytics tools provided by AWS, including Elastic Map Reduce (EMR), Data Pipeline, Elasticsearch, Kinesis, Amazon Machine Learning and QuickSight which is still in preview mode.
We will start with an overview of Data Science and Analytics concepts to give beginners the context they need to be successful in the course. The second part of the course will focus on the AWS offering for Analytics, this means, how AWS structures its portfolio in the different processes and steps of big data and data processing.
As a fundamentals course, the requirements are kept simple so you can focus on understanding the different services from AWS. But, a basic understanding of the following topics is necessary:
- As we are talking about technology and computing services, general IT knowledge is necessary, that is, the basics of programming logic, algorithms, and learning or working experience in the IT field.
- We will give you an overview of data science concepts, but if these concepts are already familiar to you, it will make your journey smoother.
- It is not mandatory but it would be helpful to have a general knowledge about AWS, most specifically about how to access your account and services such as S3 and EC2.
The following two courses from our portfolio can help you better understand the basics of AWS if you are just starting out:
- AWS Technical Fundamentals
- AWS Networking Features Essential for a Solutions Architect
If you have thoughts or suggestions for this course, please contact Cloud Academy at support@cloudacademy.com.
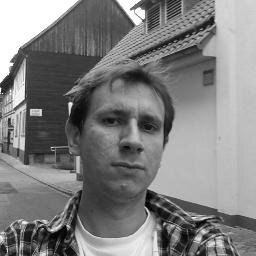
Fernando has a solid experience with infrastructure and applications management on heterogeneous environments, working with Cloud-based solutions since the beginning of the Cloud revolution. Currently at Beck et al. Services, Fernando helps enterprises to make a safe journey to the Cloud, architecting and migrating workloads from on-premises to public Cloud providers.