Explaining Concepts
This lesson explores the core concepts of machine learning, the models available, and how to train them. We’ll take a deeper look at what it means to train a machine learning model, as well as the data and methods required to do so. We’ll also provide an overview of the most common models you’re likely to encounter, and take a practical approach to understand when and how to use them to solve business problems.
In the second half of this lesson, you will be guided through a series of case studies that will show you how to apply the concepts covered in this lesson to real-life examples.
If you have any feedback relating to this lesson, feel free to contact us at support@cloudacademy.com.
Learning Objectives
- Understand the key concepts and models related to machine learning
- Learn how to use training data sets with machine learning models
- Learn how to choose the best machine learning model to suit your requirements
- Understand how machine learning concepts can be applied to real-world scenarios in property prices, health, animal classification, and marketing activites
Intended Audience
This lesson is intended for anyone who is:
- Interested in understanding machine learning models on a deeper level
- Looking to enrich their understanding of machine learning and how to use it to solve complex problems
- Looking to build a foundation for continued learning in the machine learning space and data science in general
Prerequisites
To get the most out of this lesson, you should have a general understanding of data concepts as well as some familiarity with cloud providers and their managed services, especially Amazon or Google. Some experience in data or development is preferable but not essential.
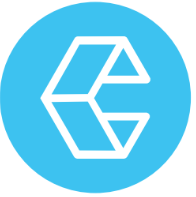
Calculated Systems was founded by experts in Hadoop, Google Cloud and AWS. Calculated Systems enables code-free capture, mapping and transformation of data in the cloud based on Apache NiFi, an open source project originally developed within the NSA. Calculated Systems accelerates time to market for new innovations while maintaining data integrity. With cloud automation tools, deep industry expertise, and experience productionalizing workloads development cycles are cut down to a fraction of their normal time. The ability to quickly develop large scale data ingestion and processing decreases the risk companies face in long development cycles. Calculated Systems is one of the industry leaders in Big Data transformation and education of these complex technologies.