Fully Connected Backpropagation
Learn about the importance of gradient descent and backpropagation, under the umbrella of Data and Machine Learning, from Cloud Academy.
From the internals of a neural net to solving problems with neural networks to understanding how they work internally, this lesson expertly covers the essentials needed to succeed in machine learning.
Learning Objective
- Understand the importance of gradient descent and backpropagation
- Be able to build your own neural network by the end of the lesson
Prerequisites
- It is recommended to complete the Introduction to Data and Machine Learning lesson before starting.
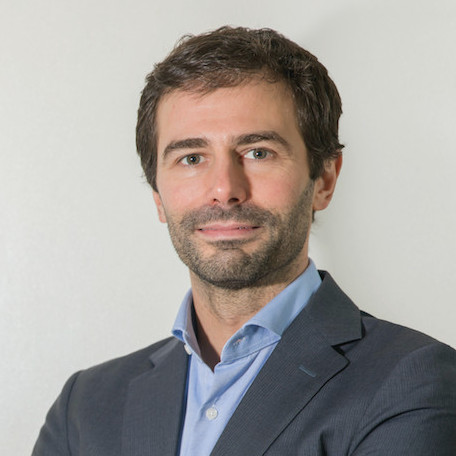
I am a Data Science consultant and trainer. With Catalit I help companies acquire skills and knowledge in data science and harness machine learning and deep learning to reach their goals. With Data Weekends I train people in machine learning, deep learning and big data analytics. I served as lead instructor in Data Science at General Assembly and The Data Incubator and I was Chief Data Officer and co-founder at Spire, a Y-Combinator-backed startup that invented the first consumer wearable device capable of continuously tracking respiration and activity. I earned a joint PhD in biophysics at University of Padua and Université de Paris VI and graduated from Singularity University summer program of 2011.