Azure Artificial Intelligence Services
This course is focused on creating practical solutions using Azure technologies in areas such as AI, messaging, the Internet of Things, and video media. This will require familiarity with dozens of Azure solutions.
This course will take you through all of the relevant technologies and ensure you know which ones to pick to solve specific problems. This course is for developers, engineering managers, and cloud architects looking to get a better understanding of Azure services.
Whether your app deals with artificial intelligence, managing IoT devices, video media, or push notifications for smartphones, Azure has an answer for every use case. This course will help you get the most out of your Azure account by preparing you to make use of many different solutions.
Learning Objectives
-
Design solutions using Azure AI technologies
-
Design solutions for IoT applications using Azure technologies
-
Create a scalable messaging infrastructure using Azure messaging technologies
- Design media solutions using Azure media technologies and file encoding
Intended Audience
-
People who want to become Azure cloud architects
Prerequisites
-
General knowledge of IT architecture
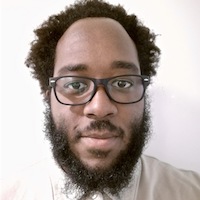
Jonathan Bethune is a senior technical consultant working with several companies including TopTal, BCG, and Instaclustr. He is an experienced devops specialist, data engineer, and software developer. Jonathan has spent years mastering the art of system automation with a variety of different cloud providers and tools. Before he became an engineer, Jonathan was a musician and teacher in New York City. Jonathan is based in Tokyo where he continues to work in technology and write for various publications in his free time.