Granularity
Power BI has changed the business intelligence (BI) landscape forever, enabling BI professionals and regular Excel users alike to work with big data and build beautiful and insightful dashboards. Integral to the Power BI platform is the data model.
This lesson discusses fundamental concepts of data modeling design within Power BI. We explore Microsoft's recommended best practices as well as common data modeling complications and the solutions to them. This lesson will prepare learners to develop data models strategically to achieve the best performance.
Learning Objectives
- Identify the primary components of data models and describe flat schemas and star schemas
- Diagnose common Power BI data modeling issues within models and recommend appropriate solutions to them
- Identify common challenges in Power BI data models, implement smart solutions, and avoid common mistakes
Intended Audience
- Business professionals whose job requires them to design and build data models in Power BI
- Anyone preparing to take the Microsoft DA-100 exam
Prerequisites
To get the most out of this lesson, you should be familiar with preparing data using Power BI.
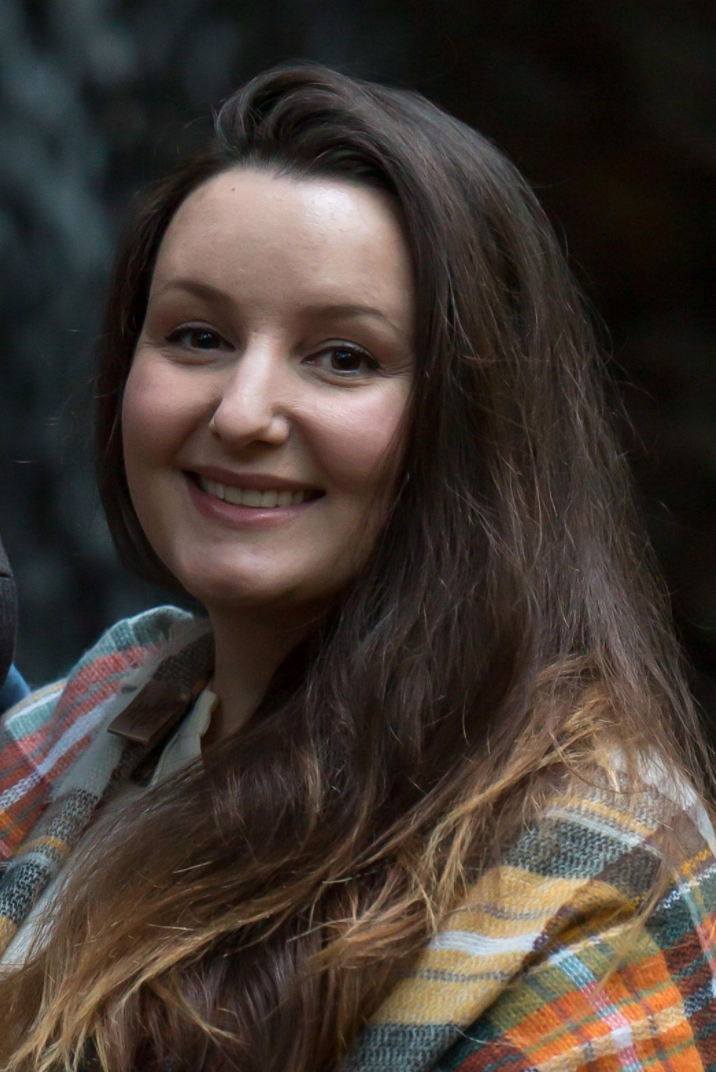
Chelsea Dohemann is a Senior Technical Trainer and Microsoft Certified Master with almost a decade of experience in technology training. She has taught an array of applications from Microsoft products including Office 365 web apps, Microsoft Office Suite, Power BI, VBA for Excel, and SharePoint to Adobe Acrobat Pro and Creative Cloud. Being a persistent learner herself, Chelsea is acutely in-tune with the challenges of learning. She presents her topics in plain language, with real-world examples, reducing complex concepts down to their simple parts.