Level 2: Release Process
Welcome to Part Two of an introduction to using Artificial Intelligence and Machine Learning. As we mentioned in part one, this lesson starts at the ground up and focuses on giving students the tools and materials they need to navigate the topic. There are several labs directly tied to this course, which will provide hands-on experience to supplement the academic knowledge provided in the lectures.
In part one we looked at how you can use out-of-the-box machine learning models to meet your needs. In this lesson, we are going to build on that and look at how you can add your own functionality to these pre-canned models. We look at ML training concepts, release processes, and how ML services are used in a commercial setting. Finally, we take a look at a case study so that you get a feel for how these concepts play out in the real world.
For any feedback relating to this lesson, please contact us at support@cloudacademy.com.
Learning Objectives
By the end of this lesson, you'll hopefully understand how to take more advanced lessons and even a springboard into handling complex tasks in your day-to-day job, whether it be a professional, student, or hobbyist environment.
Intended Audience
This lesson is a multi-part series ideal for those who are interested in understanding machine learning from a 101 perspective; starting from a very basic level and ramping up over time. If you already understand concepts such as how to train and inference a model, you may wish to skip ahead to part two or a more advanced course.
Prerequisites
It helps if you have a light data engineering or developer background as several parts of this class, particularly the labs, involve hands-on work and manipulating basic data structures and scripts. The labs all have highly detailed notes to help novice users understand them but you will be able to more easily expand at your own pace with a good baseline understanding. As we explain the core concepts, there are some prerequisites for this lesson.
It is recommended that you have a basic familiarity with one of the cloud providers, especially AWS or GCP. Azure, Oracle, and other providers also have machine learning suites but these two are the focus for this class.
If you have an interest in completing the labs for hands-on work, Python is a helpful language to understand.
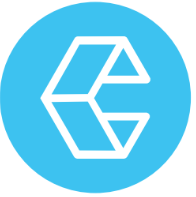
Calculated Systems was founded by experts in Hadoop, Google Cloud and AWS. Calculated Systems enables code-free capture, mapping and transformation of data in the cloud based on Apache NiFi, an open source project originally developed within the NSA. Calculated Systems accelerates time to market for new innovations while maintaining data integrity. With cloud automation tools, deep industry expertise, and experience productionalizing workloads development cycles are cut down to a fraction of their normal time. The ability to quickly develop large scale data ingestion and processing decreases the risk companies face in long development cycles. Calculated Systems is one of the industry leaders in Big Data transformation and education of these complex technologies.