Text Classification
This lesson covers the basic techniques you need to know in order to fit a Natural Language Processing Machine Learning pipeline using scikit-learn, a machine learning library for Python.
Learning Objectives
- Learn about the two main scikit-learn classes for natural language processing: CountVectorizer and TfidfVectorizer
- Learn how to create Bag-of-Words (boW) representations and TF-IDF representations
- Learn how to create a machine learning pipeline to classify BBC news articles into different categories
Intended Audience
This lesson is intended for anyone who wishes to understand how NLP works and, more particularly, how to implement it using scikit-learn.
Prerequisites
To get the most out of this lesson, you should already have an understanding of the Python programming language.
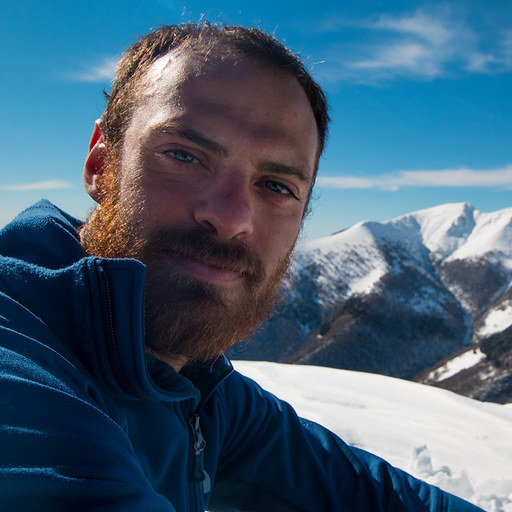
Andrea is a Data Scientist at Cloud Academy. He is passionate about statistical modeling and machine learning algorithms, especially for solving business tasks.
He holds a PhD in Statistics, and he has published in several peer-reviewed academic journals. He is also the author of the book Applied Machine Learning with Python.