Conclusion
Please note: this course is outdated and has been replaced by our Introduction to Azure Machine Learning course available here.
Machine learning is a notoriously complex subject, which usually requires a great deal of advanced math and software development skills. That’s why it’s so amazing that Azure Machine Learning Studio lets you train and deploy machine learning models without any coding, using a drag-and-drop interface. With this web-based software, you can create applications for predicting everything from customer churn rates, to image classifications, to compelling product recommendations.
In this course, you will learn the basic concepts of machine learning, and then follow hands-on examples of choosing an algorithm, running data through a model, and deploying a trained model as a predictive web service.
Learning Objectives
- Prepare data for use by an Azure Machine Learning Studio experiment
- Train a machine learning model in Azure Machine Learning Studio
- Deploy a trained model to make predictions
Intended Audience
- Anyone who is interested in machine learning
Prerequisites
- No mandatory prerequisites
- Azure account recommended (sign up for free trial at https://azure.microsoft.com/free if you don’t have an account)
This Course Includes
- 54 minutes of high-definition video
- Many hands-on demos
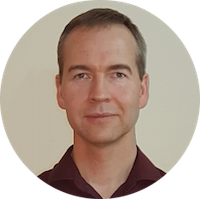
Guy launched his first training website in 1995 and he's been helping people learn IT technologies ever since. He has been a sysadmin, instructor, sales engineer, IT manager, and entrepreneur. In his most recent venture, he founded and led a cloud-based training infrastructure company that provided virtual labs for some of the largest software vendors in the world. Guy’s passion is making complex technology easy to understand. His activities outside of work have included riding an elephant and skydiving (although not at the same time).