Machine Learning Concepts
Machine learning is a hot topic these days and Google has been one of the biggest newsmakers. Google’s machine learning is being used behind the scenes every day by millions of people. When you search for an image on the web or use Google Translate on foreign language text or use voice dictation on your Android phone, you’re using machine learning. Now Google has launched AI Platform to give its customers the power to train their own neural networks.
This is a hands-on course where you can follow along with the demos using your own Google Cloud account or a trial account.
Learning Objectives
- Describe how an artificial neural network functions
- Run a simple TensorFlow program
- Train a model using a distributed cluster on AI Platform
- Increase prediction accuracy using feature engineering and hyperparameter tuning
- Deploy a trained model on AI Platform to make predictions with new data
Resources
- The GitHub repository for this course is at https://github.com/cloudacademy/aiplatform-intro.
Updates
- December 20, 2020: Completely revamped the course due to Google AI Platform replacing Cloud ML Engine and the release of TensorFlow 2.
- Nov. 16, 2018: Updated 90% of the lessons due to major changes in TensorFlow and Google Cloud ML Engine. All of the demos and code walkthroughs were completely redone.
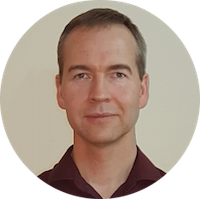
Guy launched his first training website in 1995 and he's been helping people learn IT technologies ever since. He has been a sysadmin, instructor, sales engineer, IT manager, and entrepreneur. In his most recent venture, he founded and led a cloud-based training infrastructure company that provided virtual labs for some of the largest software vendors in the world. Guy’s passion is making complex technology easy to understand. His activities outside of work have included riding an elephant and skydiving (although not at the same time).