Cluster Creation
Intermediate
17m 21s
476
4.7/5
Course Description:
GKE is Google’s container management and orchestration service that allows you to run your containers at scale. GKE provides a managed Kubernetes infrastructure that gives you the power of Kubernetes while allowing you to focus on deploying and running your container clusters.
Intended audience:
- This course is for developers or operations engineers looking to deploy containerized applications and/or services on Google’s Cloud Platform.
- Viewers should have a basic understanding of containers. Some familiarity with the Google Cloud Platform will also be helpful but is not required.
Learning Objectives:
- Describe the containerization functionality available on Google Cloud Platform.
- Create and manage a container cluster in GKE.
- Deploy your application to a GKE container cluster.
This Course Includes:
- 45 minutes of high-definition video
- Hands on demo
What You'll Learn:
- Course Intro: What to expect from this course
- GKE Platform: In this lesson, we’ll start digging into Docker, Kubernetes, and CLI.
- GKE Infrastructure: Now that we understand a bit more about the platform, we’ll get into Docker images and K8 orchestration.
- Cluster Creation: In this lesson, we’ll demo through the steps necessary to create a GKE cluster.
- Application and Service Publication: In this live demo we’ll create a Kubernetes pod.
- Cluster Management: In this lesson, we’ll discuss how you update a cluster and rights management.
- Summary: A wrap-up and summary of what we’ve learned in this course.
About the Author
Covered Topics
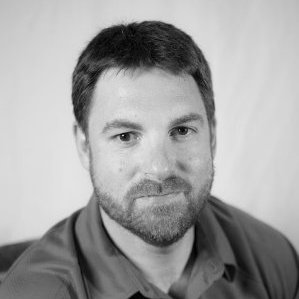
Steve Porter, opens in a new tabCloud Architect
Steve is a consulting technology leader for Slalom Atlanta, a Microsoft Regional Director, and a Google Certified Cloud Architect. His focus for the past 5+ years has been IT modernization and cloud adoption with implementations across Microsoft Azure, Google Cloud Platform, AWS, and numerous hybrid/private cloud platforms. Outside of work, Steve is an avid outdoorsman spending as much time as possible outside hiking, hunting, and fishing with his family of five.