Machine learning, with all its math and complexity, can be daunting. We’ll explore a relatively accessible technique: the Naive Bayes Classifier.
With things moving a bit more slowly through the holiday season, we’re going to re-run some of our most popular posts from 2015. Enjoy!
Machine learning can be a daunting subject. It involves involved subjects, a lot of mathematics, and sometimes emergent behavior beyond the understanding of the original implementers. This post will explore one of the easier, and more useful, machine learning techniques out there: Naive Bayes Classification.
It has been shown that we humans are quite bad at predicting outcomes, especially when there’s prior evidence. The decimals seem to scramble our brains and confuse us. Our human biases also seem to prevent us from making accurate predictions. Never mind the fact that going through documents or text and classifying it can be tedious and time-consuming. Machines, on the other hand, are not biased, do not get confused by decimals, and can do calculations much quicker than we do.
Bayes Classifier: The mathematics
A naive Bayes classifier applies Bayes’ Theorem in an attempt to suggest possible classes for any given text. To do this, it needs a number of previously classified documents of the same type. The theorem is as follows:
Bayes Classifier example: tweet sentiment analysis
As an example, let us try and find the probability that a tweet (the document) can be classified as positive (the class). At first glance the theorem can be confusing, so let’s simplify it a bit by breaking down the various components:
- P(A|B)
- This can be read as the probability of A, the class, given B, the tweet. This is the end result we’re looking for.
- P(B|A)
- This can be read as the probability of B, the tweet, given A, the class. This is determined by previously gathered information.
- P(A)
- This is the probability of A – the class. It’s independent of all other probabilities.
- P(B)
- This is the probability of B – the tweet. It’s independent of all other probabilities.
Since the probability of the tweet, P(tweet), is constant, it can be disregarded in our calculations. We’re only interested in the probability of the tweet given the class, P(tweet|positive), and the probability of the class, P(positive):
P(positive|tweet) = P(tweet|positive) * P(positive)
P(positive)
For the sake of this example, let’s say there are three possible classes: positive, negative and neutral. That gives any tweet a one in three (or 33%) chance of falling into any of those classes. That gives us P(positive) = 0.33333.
P(tweet|positive)
To calculate P(tweet|positive), we need a training set of tweets that were already classified into three categories. This gives us a basis from which to compute the probability that a tweet will fall into a specific class. Since the chances are relatively low that we’ll find a specific tweet in the training set, we’ll tokenize the tweet and calculate the probability for each word in the training set. This gives us the following formula:
P(tweet|positive) = P(T1|positive) * P(T2|positive) * .. * P(Tn|positive)
Where T1 to Tn is all the words in the tweet.
P(T1|positive)
To determine the probability of a specific word falling into the category we’re testing, we’ll need the following from the training set:
- The number of times T1 occurs in tweets that were marked as positive in the training set.
- The total number of words of tweets that were marked as positive in the training set.
There are various ways in which you can get these numbers, so we won’t go into specifics here. As an example, let’s look at the word “food”, with the following numbers:
- Number of times food occurs in positive tweets: 455
- Number of words in positive tweets: 1211
So to calculate the relative probability of food occurring in the positive category, we divide 455 by 1211, giving us 0.376. Since food can have positive, negative and neutral interpretations, it’s not surprising that its relative probability is 37%. This process now needs to be repeated for each word in the tweet.
Since we now have the ability to calculate the probabilities that each word in the tweet can be classified as positive, let’s calculate the probability that the whole tweet can be classified as positive – P(positive|tweet) = P(tweet|positive) * P(positive). For this example, let’s say the tweet was “I love good food”, and the probabilities we calculated were 25%, 62.5%, , 4% and 42.5% respectively.
P(positive|tweet) = P(tweet|positive) * P(positive)
= P(T1|positive) * .. * P(Tn|positive) * P(positive)
= 0.25 * 0.625 * 0.74 * 0.425 * 0.33
= 0.016216406
This same procedure can now be used to calculate the relative probability for each of the classes. From the training set, we calculate P(negative|tweet) as 0.000003125 and P(neutral|tweet) as 0.0082809375. Once we have the probability for each class, we can compare the classes, and use the highest ranked class as the class for the document. Intuitively, it makes sense to classify I love good food as positive, but now we have a mathematical proof, based on gathered data, that it can be classified as positive.
Bayes Classifier: some considerations
When you read up on the Bayes classifier, you’ll see that it’s often called the Naive Bayes classifier. It’s called naive because the classifier assumes that the document and their words are independent of each other. This assumption greatly simplifies and at the same time speeds up the needed calculations, but reduces the classifier’s accuracy. Despite this reduced accuracy, the classifier is still surprisingly accurate, and fast to boot.
There are some features of the theorem or the data set that can severely skew the calculated probabilities. On the one hand, the repeated use of decimals can result in very low numbers, sometimes interpreted as zero, on computers. This is known as underflow. On the other hand, if we try to calculate the probability for a word that doesn’t exist in the training set, it will come out as zero. Since the final probability is the product of the probabilities of all the words, this will result in a final probability of zero as well, regardless of how high, or low, the other probabilities are. To prevent this from happening, we apply a technique called smoothing. Using these techniques will greatly increase the accuracy of your classifier.
Bayes Classifier: implementation
It’s relatively easy to find an implementation of the Bayes classifier in your language of choice. A couple of examples are the classifier gem for Ruby, and the NLP package for PHP. The code below shows the classification of the tweet we’ve just discussed using a previously defined training set and the classifier gem:
require 'classifier' # Set up the classifier classifier = Classifier::Bayes.new('Positive', 'Neutral', 'Negative') # Train the classifier CSV.foreach('training_set.csv') do |row| # In the format category,tweet classifier.train(row[0], row[1]) end # Use the classifier b.classify 'I love good food' # Returns "Positive"
You may also find this dataset useful for experimenting on your own.
Summary
Despite all the complicated mathematics, implementing a Bayes classifier is all about counting the number of words, documents and categories. Once you have these, you can combine them to calculate the probability for each of the possible classes. The document is then classified according to the highest calculated probability. Although there are some factors to take into consideration when using the Bayes filter, in general, it should prove to be a profitable and easy first step into Machine Learning.
If you’d like to understand more Machine Learning, visit Cloud Academy’s Machine Learning training library.
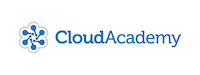
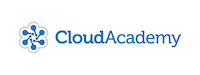