Machine Learning Algorithms
Advanced
5m 41s
2,985
4.4/5
When we saw how incredibly popular our blog post on Amazon Machine Learning was, we asked data and code guru James Counts to create this fantastic in-depth introduction to the principles and practice of Amazon Machine Learning so we could completely satisfy the demand for ML guidance within AWS.
If you've got a real-world need to apply predictive analysis to large data sources - for fraud detection or customer churn analysis, perhaps - then this course has everything you'll need to know to get you going.
James has got the subject completely covered:
- What exactly machine learning can do
- Why and when you should use it
- Working with data sources
- Manipulating data within Amazon Machine Learning to ensure a successful model
- Working with machine learning models
- Generating accurate predictions
About the Author
Covered Topics
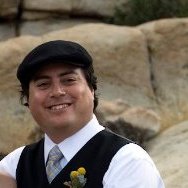
James Counts, opens in a new tabSoftware Developer
James is most happy when creating or fixing code. He tries to learn more and stay up to date with recent industry developments.
James recently completed his Master’s Degree in Computer Science and enjoys attending or speaking at community events like CodeCamps or user groups.
He is also a regular contributor to the ApprovalTests.net open source projects, and is the author of the C++ and Perl ports of that library.