The growing pressures of dealing with data at massive scale require purpose-built solutions. Meet Apache Storm and Apache Kafka.
In our hyper-connected world, countless sources generate real-time information 24 hours per day. Rich streams of data pour in from logs, Twitter trends, financial transactions, factory floors, click streams, and much more, and developing the ability to properly handle such volumes of high-velocity and time-sensitive data demands special attention. Apache Storm and Kafka can process very high volumes of real-time data in a distributed environment with a fault-tolerant manner.
Who Uses Storm and Kafka – And Why You Should Care
Both Storm and Kafka are top-level Apache projects currently used by various big data and cloud vendors. While Apache Storm offers highly scalable stream processing, Kafka handles messages at scale. In this post, we’ll see how both technologies work seamlessly together to form the bedrock of your real-time data analysis pipeline. You’re going to learn the basics of Apache Storm how to quickly install it in the AWS cloud environment.
A Gentle Introduction to the Apache Storm Project
By design, Apache Storm is very similar to any other distributed computing framework like Hadoop. However, while Hadoop is used for batch or archival data processing, Storm provides a framework for streaming data processing. Here are the components of a Storm cluster:
(Storm cluster components)
Nimbus Node
The Nimbus Service runs on the master node (Like Job Tracker in Hadoop). The task of Nimbus is to distribute code around the cluster, assign tasks to servers, and monitor for cluster failures.
ZooKeeper Nodes
Nimbus relies on the Apache ZooKeeper service to monitor message processing tasks. The worker nodes update their task status in Apache ZooKeeper.
Supervisor Nodes
The worker nodes that do the actual processing run a daemon called Supervisor. Supervisor receives and manages worker processes to complete the tasks assigned by Nimbus.
There are a few more Apache Storm-related terms with which we should be familiar before we actually get started:
- Topology: The workflow or the computation job graph is called topology. Much like MapReduce jobs in Hadoop, Apache Storm uses topologies. Each node in a topology has specific tasks, and the link between nodes draws the graph of how computational jobs flow from source to final output.
- Tuple. A unit of data.
- Streams. Unbounded sequences of tuples. Streams are raw information sources that are processed by Spouts and Bolts to generate specific output. For example, a Twitter Stream could be processed by Spouts and Bolts to generate top trending topics.
- Spouts. A Spout is the source of a stream. In our earlier example, a spout is connected to a Twitter API to capture a stream of tweets. Alternatively, a Spout can be connected to a messaging system to read incoming messages and pass them forward to be processed by Bolts.
- Bolts. Bolts consume input from Spouts and produce outputs or input for another Bolt. Bolts are the workers in the Storm topology, containing the smallest units of processing logic in a topology. Bolts run functions, filter, aggregate or join data, filter tuples and talk to data stores like databases.
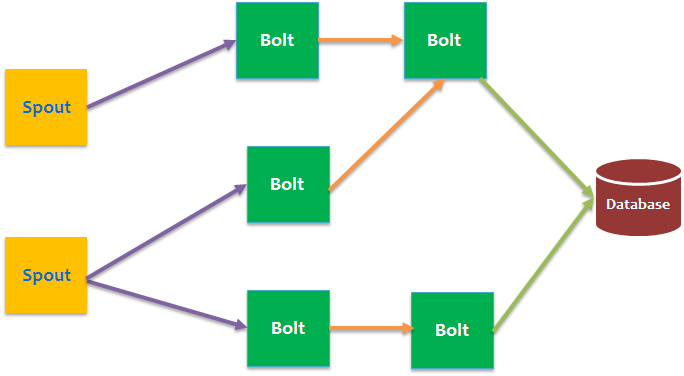
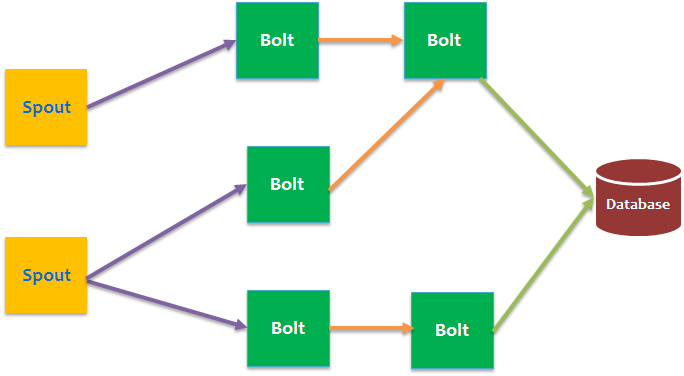
(Storm topology)
Manually Deploying Apache Storm on AWS
Setting up Apache Storm in AWS (or on any virtual computing platform) should be as easy as downloading and configuring Storm and a ZooKeeper cluster. The Apache Storm documentation provides excellent guidance. In this blog post, however, we’re going to focus on storm-deploy – an easy to use tool that automates the deployment process.
Deploying Apache Storm on AWS using Storm-Deploy
Deploying with storm-deploy is really easy. Storm-deploy is a github project developed by Nathan Martz, the creator of Apache Storm. Storm-deploy automates both provisioning and deployment. In addition, it installs the Ganglia interface for monitoring disk, CPU, and network usage. I will assume that you already have an AWS account with privileges for AWS EC2 and S3.
You will need to install a Java version greater than jdk-1.6 from your workstation. Storm-deploy is built on top of jclouds and pallet. Apache jclouds is an open-source, multi-cloud toolkit for the Java platform that lets you create applications that are portable across clouds. Similarly, Pallet is used to provision and maintain servers on cloud and virtual machine infrastructure, by providing a consistently configured running image across a range of clouds. It is designed for use from the Clojure REPL (Read-Eval-Print Loop), from Clojure code, and from the command line. I simply followed the storm-deploy github project documentation. The commands are universal.
Now let’s see how all this is really done.
Step-1: Generate password-less key pairs:
ssh-keygen -t rsa
Step-2: Install leningen-2. Leiningen is used for automating Clojure project tasks such as project creation and dependency downloads. All you will need to do here is to download the script:
wget https://raw.github.com/technomancy/leiningen/stable/bin/lein
Step-3: Place the script it on your path, say /usr/local/bin, and make it executable.
chmod +x /usr/local/bin/lein
Step-4: Clone storm-deploy using git.
git clone https://github.com/nathanmarz/storm-deploy.git
Step-5: Download all dependencies by running:.
lein deps
Step-6: Create a ~/.pallet/config.clj file in your home directory and configure it with the credentials and details necessary to launch and configure instances on AWS.
(defpallet :services { :default { :blobstore-provider "aws-s3" :provider "aws-ec2" :environment {:user {:username "storm" ; this must be "storm" :private-key-path "$YOUR_PRIVATE_KEY_PATH$" :public-key-path "$YOUR_PUBLIC_KEY_PATH$"} :aws-user-id "$YOUR_USER_ID$"} :identity "$YOUR_AWS_ACCESS_KEY$" :credential "$YOUR_AWS_ACCESS_KEY_SECRET$" :jclouds.regions "$YOUR_AWS_REGION$" } })
The parameters are as follows:
- PRIVATE_KEY_PATH: Path to private rsa key, e.g. ~/.ssh/id_rsa.
- PUBLIC_KEY_PATH: Path to public rsa key, e.g. ~/.ssh/id_rsa.pub. For Linux, you should have a null passphrase on the keys.
- AWS_ACCOUNT_ID: Can be found on the AWS site, under Security Credentials and Account Identifiers. The ID uses the format XXXX-XXXX-XXXX.
- AWS_ACCESS_KEY: Your AWS Access Key.
- AWS_ACCESS_KEY_SECRET: Your AWS Access Secret Key. This can be downloaded from the AWS IAM dashboard.
- AWS_REGION: The region in which the instances will be launched.
Step-7: There are two very important configurable files. Here the path is for my set up: /opt/chandandata/storm-cluster/conf/clusters.yaml. The first file, clusters.yaml, looks like this:
################################################################################ # CLUSTERS CONFIG FILE ################################################################################ nimbus.image: "us-east-1/ami-d726abbe" #64-bit ubuntu nimbus.hardware: "m1.large" supervisor.count: 2 supervisor.image: "us-east-1/ami-d726abbe" #64-bit ubuntu on eu-east-1 supervisor.hardware: "m1.large" #supervisor.spot.price: 1.60 zookeeper.count: 1 zookeeper.image: "us-east-1/ami-d726abbe" #64-bit ubuntu zookeeper.hardware: "m1.large"
All of the properties are self-explanatory. We have set the cluster to lunch instances in us-east-1 with an AMI ID: ami-d726abbe and an instance size of m1.large. (This might be an odd configuration file. It is normally better to use m3 or m4 series instances). You can configure the hardware and images and select a spot pricing maximum – if that’s what you want.
Step-8: The other file, storm.yaml, is where you can set storm-specific configurations.
Step-9: Launch the cluster:
lein deploy-storm --start --name cluster-name [--branch {branch}] [--commit {commit tag-or-sha1}]
- The –name parameter names your cluster so that you can attach to it or stop it later. If you omit –name, it will default to “dev”. The name must be in lower-case.
- The –branch parameter indicates which branch of Storm to install. If you omit –branch, it will install Storm from the master branch.
- The —commit parameter allows a release tag or commit SHA1 to be passed. If you omit –commit you will get the latest commit from the branch you are using.
- Example: lein deploy-storm –start –name cp-cluster –branch master –commit 0.9.1
- This will also install with the latest version:
lein deploy-storm --start --name cp-cluster
- The deploy sets up Nimbus, the Supervisors, and ZooKeeper launches the Storm UI on port 8080 on Nimbus and launches a DRPC server on port 3772 on Nimbus.
- In case of errors, log into the instances with ssh storm@<IP_OF_MACHINE>, and check the logs in ~/storm/logs.
This will run the cluster with appropriate settings. Before submitting an actual storm job, you should know about just a few additional tasks that can be performed.
Operations on an Apache Storm Cluster
- To attach to an Apache Storm cluster, run the following:
lein deploy-storm --attach --name cp-cluster
Attaching to a cluster configures your storm client (which is used to start and stop topologies) to talk to that particular cluster as well as giving your workstation authorization to view the Storm UI on port 8080 on Nimbus. It writes the location of Nimbus in ~/.storm/storm.yaml so that the storm client knows which cluster to talk to, and authorizes your workstation to access the Nimbus daemon’s Thrift port (which is used for submitting topologies), and to access Ganglia on port 80 on Nimbus.
- To get the IP addresses of the cluster nodes, run the following:
lein deploy-storm --ips --name mycluster
- To access the ganglia browser UI, go to: http://{nimbus ip}/ganglia/index.php
- To stop the cluster, run:
lein deploy-storm --stop --name cp-cluster
Submit an Apache Storm Job
You can download Apache Storm here. At the time of this writing, the latest Storm version was 0.9.6. We have attached our workstation to the storm cluster so we are ready to run a Storm job.
- Go to storm-0.9.6/bin and run:
storm jar PATH_TO_JAR.JAR JOB_CLASSNAME
- Check the storm UI <NIMBUS_HOST:8080> to check the job status.
- To kill the job, run:
storm kill TOPOLOGY_NAME
Next Steps: Harnessing the Combined Power of Storm and Kafka
In this post, you’ve been introduced to Apache Storm and how to easily deploy a storm cluster using storm-deploy. But as I mentioned earlier, Storm on its own is not capable of large scale real-time data processing. We need to add Kafka, which acts as the distributed messaging service for storm topology. We will discuss Kafka integration and compare it with Amazon Kinesis in a later post. Stay tuned!
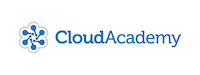
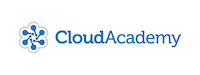