How to apply Machine Learning to social media to make your customers happy
At his AWS re:Invent presentation, Alex Ingerman – technical product manager at AWS – went through the design and implementation of a real-world end-to-end application to transform a high-volume social stream into actionable support requests for your customer support team.
The idea is that social networks like Twitter generate a huge amount of conversations, but only a very small percentage are actually useful and require some follow-up action. By means of smart automation, we can easily and efficiently filter out all the noise (i.e. about 80% of the stream), with the ultimate goal of making our customers happy.
The proposed software pipeline makes use of five AWS services: Amazon Machine Learning, Kinesis, Lambda, SNS, and Mechanical Turk.
Amazon Machine Learning will be used to classify tweets into either actionable or not actionable requests by means of a binary classifier, while Mechanical Turk will be used to label our tweets quickly and cheaply. The remaining services will provide the whole system’s logic, which can be abstracted into a more general framework to handle many more use cases and scenarios.
How do you train your Machine Learning model?
In order to build a useful classification model, we need a labeled dataset of tweets. We can easily retrieve huge numbers of tweets using the official Twitter APIs, although we would still need to manually label them. This is where AWS Mechanical Turk can help us automate the labeling process and create a custom MT task. A few thousand tweets can be classified within hours and at a fraction of the cost.
Once we have a real dataset, we can split it into a training and a testing set (i.e. two DataSource objects on AmazonML). These objects will provide us with interesting statistics about our dataset, and we can dig a bit deeper before proceeding with creating our model. For example, we may discover that removing duplicated tweets and retweets from our dataset would result in a cheaper labeling phase and faster training.
As part of the presentation, Alex showed us how to create DataSource objects and how to create a new model using Python (with the boto3 library).
As soon as the model is trained, we can enable it for real-time predictions and, based on the business impact of eventual errors, incrementally adjust its behavior.
The basic assumption is that every predictive model makes mistakes and therefore we always have to find the best trade-off between false positives and false negatives, based on our business goals and the cost of a mistake. In this specific case, false negatives are much worse than false positives, since ignoring a real problem is much more costly than manually handling a non-actionable tweet.
AWS Lambda and Kinesis for streaming data processing
We can send our stream of social activities to AWS Kinesis and connect it to AWS Lambda, which will take care of the core application logic. Basically, Lambda will interact with our Machine Learning model to understand if a tweet is actionable or not, and then either discard it or forward it to a customer support agent via SNS (via email, for example).
Our model will also return a confidence measure, allowing us to take more advanced actions based on its value. We could also add more complexity by labeling our dataset with more granular data, for example about the request type (i.e. “technical question”, “support request”, “problem report”, “feature request”) and training a multi-class model to send each tweet to the right support team.
Conclusions
This application can be seen as a general pattern to tackle many different problems, whenever you have a streaming data source, a per-instance processing task, a classification model, and a notification output.
The full source code of this application is available here. You can view the slides from the presentation here.
If you want to get a jump start on Amazon Machine Learning, check out Cloud Academy’s Machine Learning Learning Path.
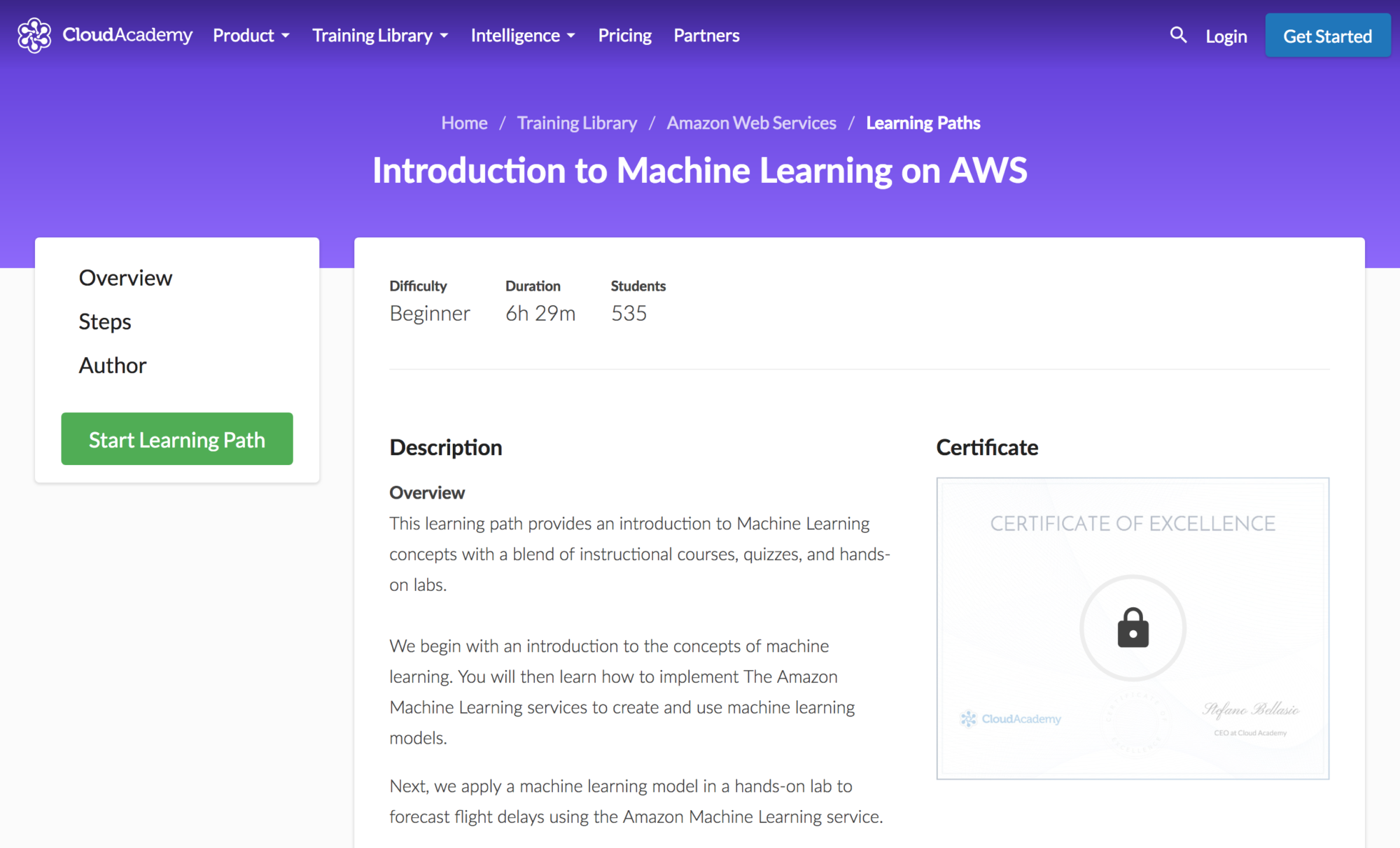
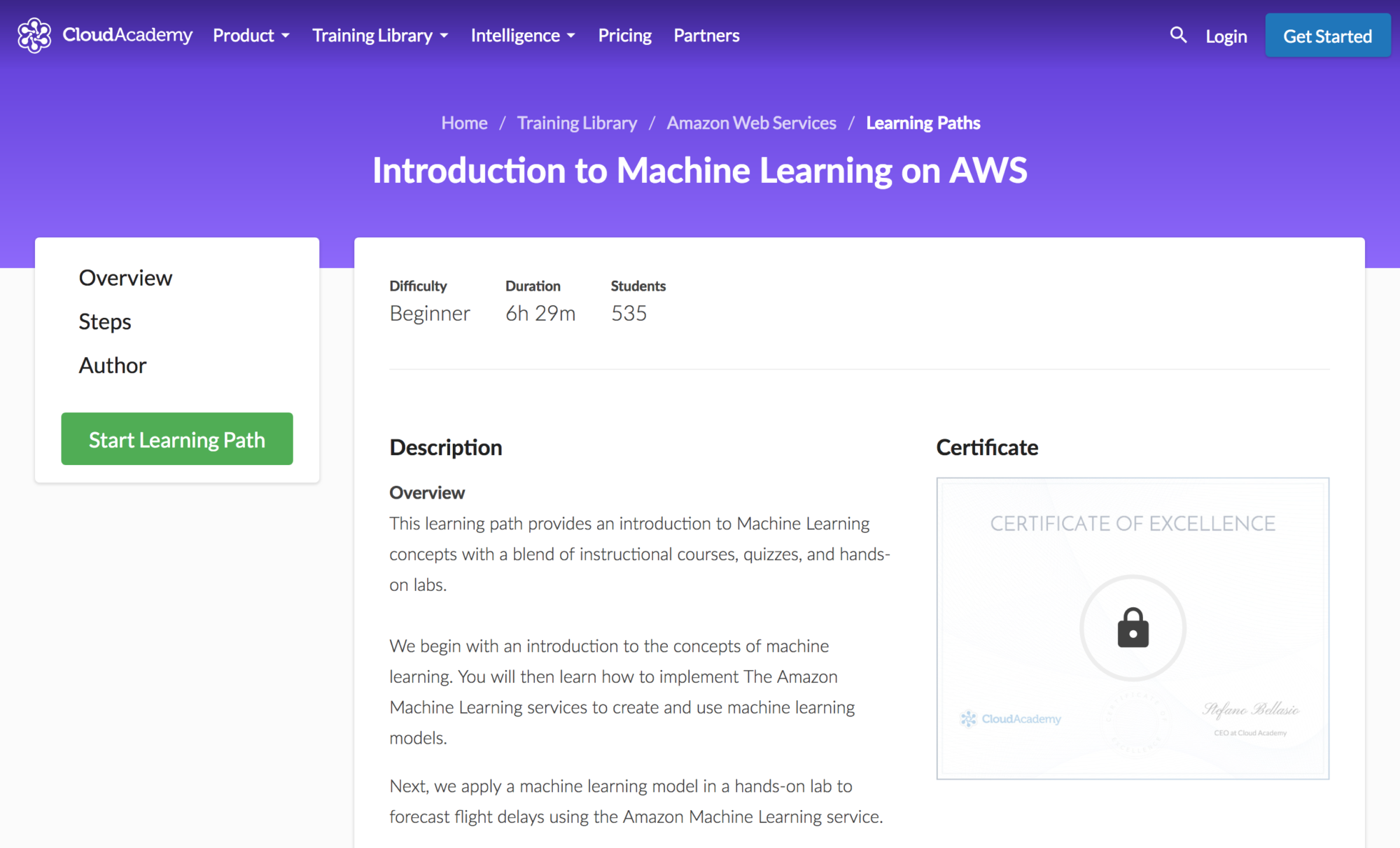
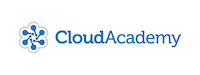
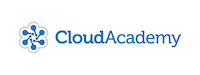